IBM Watsonx: Where AI Meets Human Wisdom in Retail
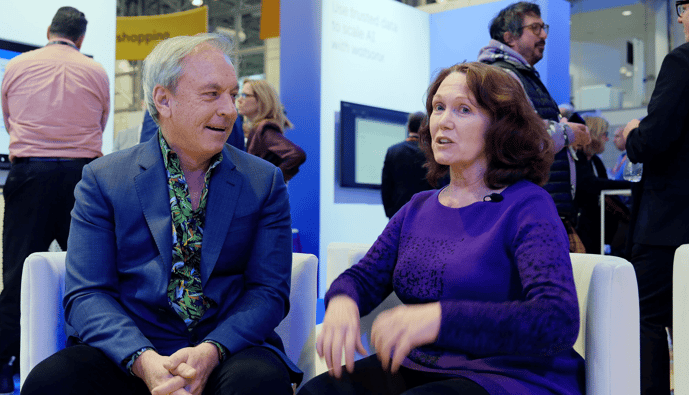
Subscribe to our newsletter
I enjoyed being immersed in IBM’s Watsonx at the National Retail Federation’s Big Show last month. The crux of what IBM Watsonx is all about is keeping the humanness of this new kind of smart computer technology called generative AI.
My colleague Neil Saunders said, “The challenge [with AI in retail] lies in finding the use cases that will actually be revolutionary and separating it from those that are mere flights of fantasy. In many cases, retail remains a very human-centric business and that should not be forgotten.”
The important distinction is knowing the difference between artificial intelligence (AI), which has been around for a while, and generative AI. The former has been around for many years and can take big data sources and find patterns. The breakthrough Watson showed the world over a decade ago was that it could take vast amounts of data, find the patterns, and generate new responses.Many have touted how an individual could use generative AI to create customized ad content, email subjects, and product descriptions that could bring a unique touch to each shopper’s journey.
Generative AI is in the early stages at the consumer level, but IBM has been using artificial intelligence to support large enterprises and their digital transformation for years.
Nancy Greco, Global Tech Sales, Distribution, and IBM Distinguished Engineer, shared that there is always a human checking IBM’s Watsonx generative AI so it doesn’t hallucinate because generative AI is most powerful when combined with human evaluation, feedback, and decision-making. It is not a stand-alone product or technology.
Before I get too deep in the weeds, let me offer my explanation of generative AI because many people either dismiss it or fear it. Those people can become paralyzed by fear and not see the innovation and possibilities. They only see that they will be replaced.
Consider this…
You’ve seen those cooking shows where everything is pre-cut for the chef. From the rinsed, chopped, and sorted lettuces to the exact 1/8 teaspoon of Cumin, all set out in bowls so the chef can create. “With generative AI,” Greco said, “you are the chef. It opens so many possibilities.”
Four main buckets are needed to get the right results from your data via Generative AI. I’ll stay with the cooking analogy.
The Data Set: Your Ingredients
Think of your data like the ingredients in a recipe. This could be shopper behavior data, product specifications, or other retailer metrics. Often, retailers find their data incomplete, like missing ingredients in a recipe. They might need to purchase or blend additional data with what they already have. This step is crucial to paint a full picture for the generative AI to work with, much like ensuring you have all the right ingredients before you start cooking.
Generative AI: The Chef
Generative AI is like a master chef who can handle far more ingredients (or data points) than any human could. But here's the catch: it needs to be trained by humans on what to focus on. Just like a chef needs to know which flavors to combine, the AI needs guidance on which important data points to review from their library of thousands of large-scale AI models trained on language, geospatial data, IT events, and code. This training allows the AI to access and interpret vast data sets effectively.
Prompts: The Recipe
Think of prompts as the recipe our generative AI chef follows. Crafting these prompts is a skill, almost like being a sous chef. People are carving careers out of being prompt experts. However, the need for specialized prompt creators might diminish as generative AI evolves. The AI will become more adept at understanding and executing complex instructions, much like a chef becoming proficient in preparing dishes without needing a detailed recipe.
Trust and Governance: Quality Control
Governance is your quality control in our kitchen analogy. This is where IBM shines. They ensure that Watsonx’s output is free from biases, hate speech, or any other undesirable elements, similar to ensuring the food is safe and meets health standards. For instance, consider a scenario where a mattress company uses AI to write an email campaign. If the generative AI inadvertently mimics a unique style from a public figure like Sarah Silverman, drawn from its data set, it could lead to copyright issues. This highlights the importance of governance in overseeing the AI's output and ensuring legal and ethical compliance.
So many people used AI as a buzzword at NRF. I wanted to hear IBM tell me what unique problem their customers are trying to solve with generative AI, specifically, focusing on solving problems with a quantifiable return on investment.
According to a recent study published by IBM’s Institute for Business Value, consumers want AI to simplify the shopping journey. Roughly four in five consumers who haven’t yet used artificial intelligence for shopping would like to see how it can help them research products or get product information (86%), look for deals and promotions (79%) or get service, ask questions, and resolve issues (82%).
42% of retail CEOs and 59% of consumer products CEOs expect AI—including gen AI, deep learning, and machine learning—to be the technology that can best deliver desired results in the next three years. Why? Because of all the ways it can make shopping easier.
The Italian clothing company Max Mara is an example of how technology aided a retailer.
For Max Mara, like many other companies, the pandemic’s arrival—and the changes in buying behavior it produced—accelerated a digital transformation that had already been underway. In fact, during the pandemic, the digital share of business volume nearly tripled. As if crossing a threshold, Max Mara’s digital operations unit—established early in its digital transformation journey—recognized that the efficiency of its back-end operations would now have an even larger impact on customer satisfaction.
Himanshu Shrivastava shared at an IBM fireside chat that they keep loyalty with their Al-Futtaim Group customers in India by being the resource for pretty much anything. They have over 4 to 5,000,000 registered users of their eight or nine million customers on the app, and they are finding that people can use the app to start their own businesses and access those customers. All powered with AI, they are looking at how generative AI will help their app be the one source for everything: search, services, omnichannel purchases—the works.
I can see a world where a women’s wear buyer could predict her best sellers by simply asking her computer.
The only way to do that now is a seasoned buyer and tracking skus for, let's say, “black cocktail dresses.”
The problem with doing that now is that you don't know what length will sell. What material? What was the previous sell-through at 14 days in-store? What sizes? What discounts? How about the country it was made in? Where were the goods sourced? What was the sleeve style? How many were returned? For what reason: online or in-store? How many went to a third party to clear?
Many retailers have sophisticated algorithms to help them, but those analyze the past - and on a limited basis. The information is usually locked into silos - marketing, finance, etc.
Generative AI could generate the best seller for 2025 based on all those data points and even the weather, unemployment rate, rural versus city locations, etc.
Instead of sending out a general “take 20% off” email, you could send a truly personal email or deliver a social media ad tailored to one person, showing three looks they would love and have to come into the store to see.
See what else is possible in this video below with Nancy Greco, me, and Tony Drockton, founder and CEO of Hammitt.
Smart retailers will gather all the data they can on their products to produce those amazing results.
Generative AI is more than creating a pretty email or a funny picture of a fish driving a car—much more than that.
#sponsoredpost